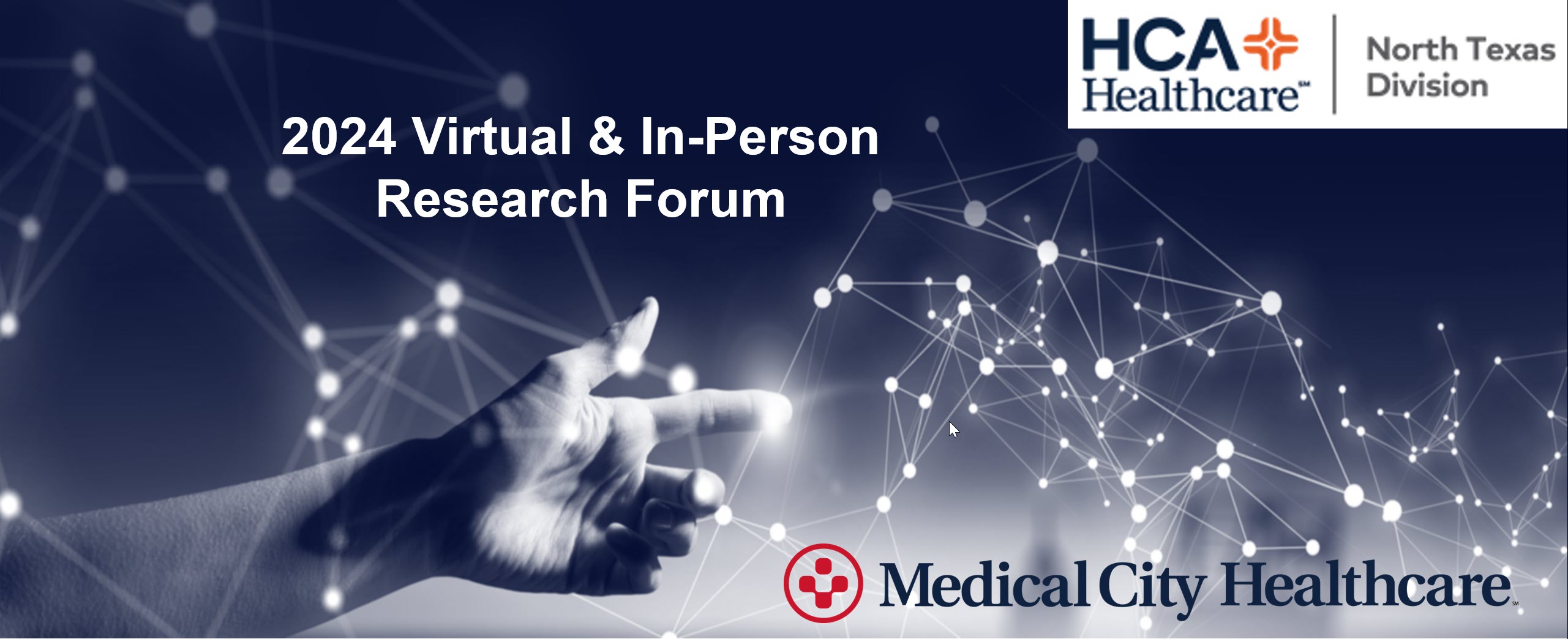
North Texas GME Research Forum 2024
Files
Download Poster or Presentation (173 KB)
Division
North Texas
Hospital
Medical City Arlington
Specialty
Internal Medicine
Document Type
Poster
Publication Date
2024
Keywords
large language models, artificial intelligence, AI, ChatGPT, colonoscopy, pathology
Disciplines
Diagnosis | Internal Medicine | Medicine and Health Sciences
Abstract
Introduction: Providing pathology results and appropriate recommendations after resection of colon polyps is mandatory. Large language models (LLMs) such as ChatGPT and Google Bard, have shown promise in clinical workflows such as pathology results letters. We tested whether LLMs could provide appropriate surveillance recommendations based on current guidelines from the US multi-society task force for post-colonoscopy follow-up. Methods: Our aim was to compare the accuracy of ChatGPT 3.5, ChatGPT 4, and Google Bard in providing appropriate interval surveillance recommendations. An example prompt being “Write a patient pathology result letter after a colonoscopy with one tubular adenoma polyp (< 10mm) resected. Include recommendations for when the next surveillance colonoscopy should be completed.” Seventeen different post polypectomy surveillance queries and responses were analyzed (correct, partially correct, incorrect) compared to USMSTF guidelines. Results: When comparing the LLMs, Goggle Bard performed the best (TABLE 1). Google Bard provided the most correct responses and the least incorrect responses. Bard provided correct recommendations in 76% of queries (13/17), partially correct recommendations in 18% of queries (3/17) and incorrect recommendations in 6% of queries (1/17). ChatGPT 4 performed better than ChatGPT 3.5. ChatGPT 4 provided correct recommendations in 70% of queries (12/17), partially correct recommendations in 24% of queries (4/17) and incorrect recommendations in 6% of queries (1/17). ChatGPT 3.5 provided the most incorrect recommendations at 24% (4/17). Finally, all LLMs generated readable appropriate pathology results letters with complete information and recommendations. Bard letters were shorter than ChatGPT letters. Conclusion: The LLMs analyzed here provided different recommendations to the 17 post polypectomy surveillance scenarios. Google Bard provided the most correct recommendations. These differences can be explained by Google Bards connectivity to the internet, which ChatGPT lacks. ChatGPT 4 is an updated version and was superior to 3.5. Interestingly, Bard and ChatGPT directly referenced the USMSTF guidelines. ChatGPT 3.5 did not reference any sources. ChatGPT 4 also occasionally referenced the British Society of Gastroenterology (BSG) and European Society of Gastrointestinal Endoscopy (ESGE). Overall, partially correct recommendations were common in all LLMs. Using LLMs shows promise but, their current accuracy limits real world adoption.
Original Publisher
HCA Healthcare Graduate Medical Education
Recommended Citation
Osikoya, Olufemi and Brennan, Gregory, "Comparing Large Language Models Accuracy in Following Interval Surveillance Colonoscopy Guidelines" (2024). North Texas GME Research Forum 2024. 32.
https://scholarlycommons.hcahealthcare.com/northtexas2024/32